Are you intrigued by data science buzzwords but unsure of their actual meaning or application in the industry? Maybe you’re an emerging data analyst, trying to get a handle on the various visualization tools out there. This guide hopes to discuss one. In this Content, we hope to begin a path to understanding a box plot.
Unlocking the Structure: Anatomy of a Box Plot
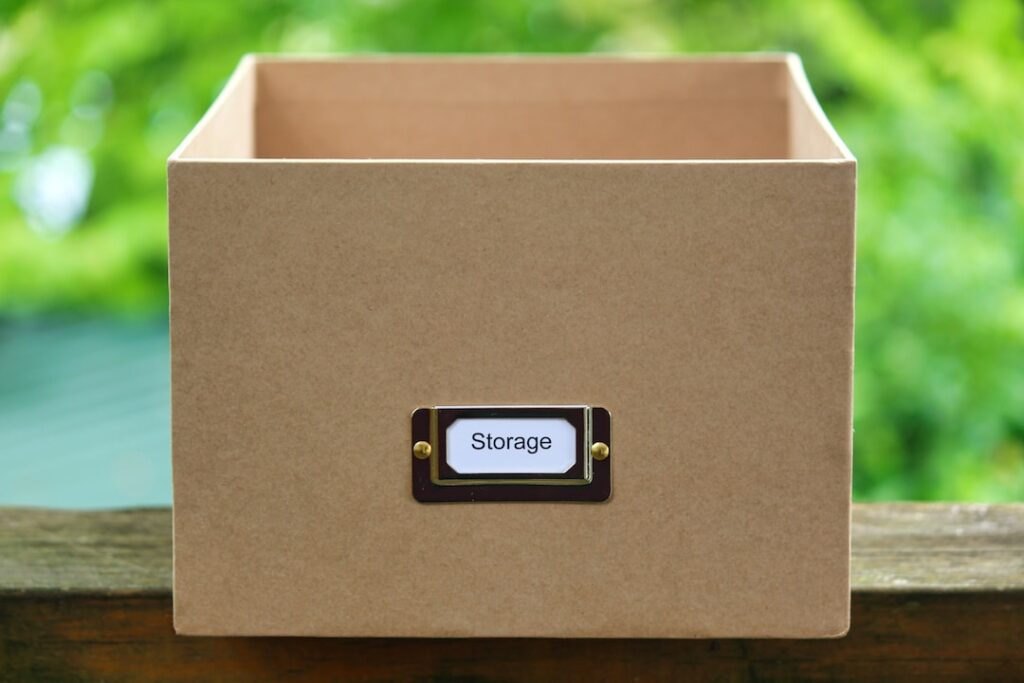
The initial step in delving into the nuances of a box plot is the identification of its components. So, what does a box plot consist of? Essentially, it comprises a box (hence the name), which encases the IQR (from Q1 to Q3), and the ‘whiskers’ extending from the box indicating variability outside the upper and lower quartiles.
The ‘box’ sets on the left the first quartile (Q1), which represents the 25th percentile of the data, and on the right the third quartile (Q3), the 75th percentile. The line inside the box is the median or the second quartile (Q2) and marks the 50th percentile of the data set.
The whiskers are lines that extend from the box to the highest and lowest observations, excluding outliers. The concept of outliers is relative and context-dependent, but commonly any value that falls beyond 1.5 times the IQR above the third quartile or below the first quartile is defined as an outlier.
In some cases, box plots can be extended to include an additional feature, a notch. A notch placed around the median is purposed to visually assess the significance of the difference between medians.
How To Interpret Box Plots for Powerful Data Insights
Interpreting a box plot might appear daunting at first, but it’s straightforward when you understand its structure. A classic box plot holds substantial information pertaining to the dataset it represents.
The median (Q2) gives us the ‘central tendency’, which is indicative of a ‘typical’ value in the dataset. The range between Q1 and Q3 (the IQR) points towards the variability of the dataset, which holds 50% of the values.
Furthermore, the length of the whiskers gives insight into the spread of the data points within the dataset. If the whiskers stretch far, it means a great deal of variability; conversely, if they’re short, it signifies little spread.
Lastly, outliers, if any, can be visualized as individual points that are separate from the box-and-whisker construct, indicating anomalies or surprising values within the dataset. The presence of outliers often prompts analysts to dig deeper for their cause.
The Role of Box Plots in Outlier Detection
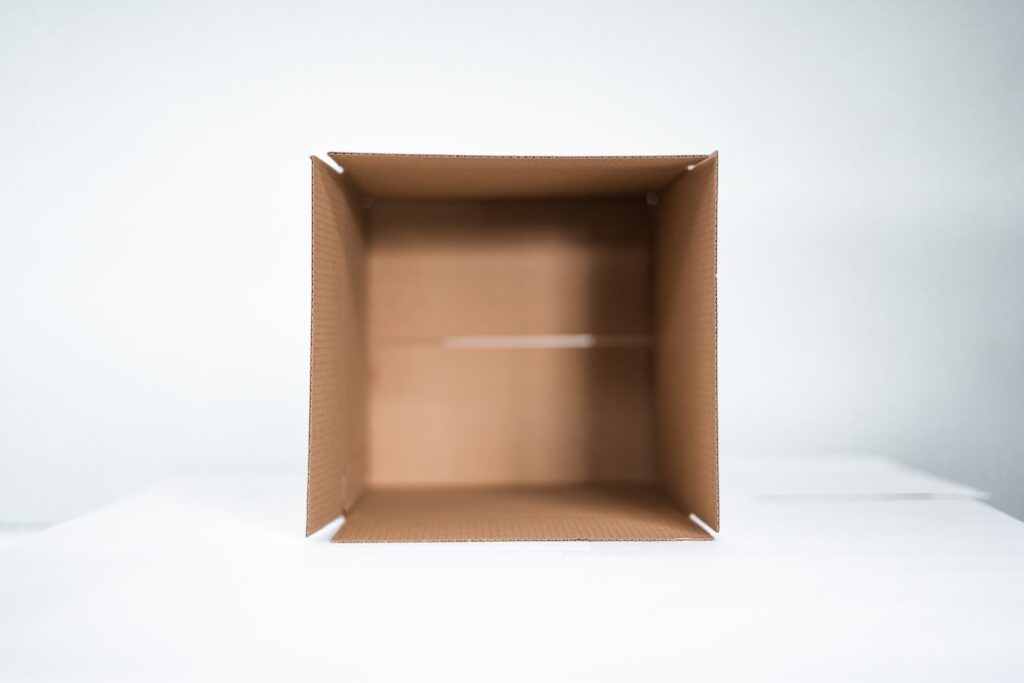
Probably the most significant benefit of using a box plot is its capacity for outlier detection. Due to their structure, box plots are well-suited to identify and illustrate outliers within datasets.
An outlier is often any data point that lies over 1.5 times the interquartile range above the upper quartile or below the lower quartile. In the context of a box plot, outliers are present as tiny circles or dots beyond the whiskers.
Finding these outliers is critical as they can significantly skew and mislead the training process in data modeling, resulting in considerably less accurate models. Through a box plot, these outliers can be easily detected and considered in the data processing and crafting of accurate, reliable models.
Box plots are an essential data analytics tool for digesting large datasets into a digestible structure. With their five-number summary and ability to visualize outliers, they allow for a comprehensive understanding of data dynamics, ultimately empowering decisive, fact-driven actions.